People don’t realise what powers their Chat GPT conversations. A massive network of data centres makes these Artificial Intelligence interactions possible. The United Kingdom has 404 facilities across 76 markets, while Australia operates 252 data centres in 26 markets.
These numbers tell just part of the story about power consumption. London’s 141 data centres in one city show the concentrated scale of AI infrastructure. Australia’s facilities are spread over 7.5 million square kilometres, which demonstrates the country’s geographic reach. The sheer size of the infrastructure needed to keep AI systems running raises concerns. More AI services mean more data centres, which leads to questions about how our digital conversations affect the environment.
Let’s examine the actual energy costs that drive Chat GPT’s operations. We’ll also examine the huge data centre network behind it and see how our growing dependence on AI technology affects our environment.
The Massive Data Centres Behind Chat GPT
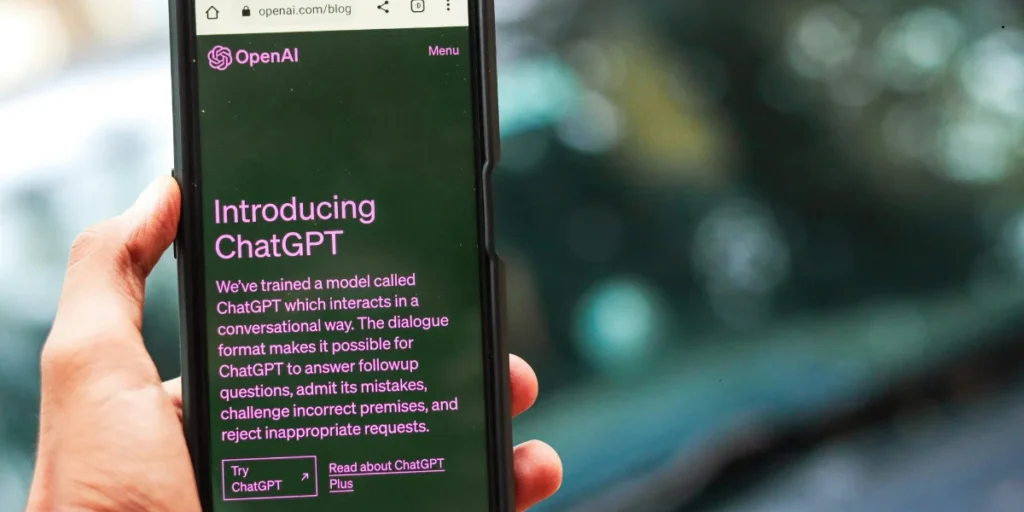
Chat GPT’s backend relies on a vast network of specialised hardware in massive data centres. Microsoft Azure’s infrastructure powers Chat GPT through more than 7,500 virtual machines that process user prompts.
These AI systems need extraordinary computational power. Training large language models takes hundreds or thousands of expensive GPUs working together in data centres for weeks or months. For example, BLOOM, a 176-billion parameter system like GPT-3, needed 117 days of training on a 384-GPU cluster. Google’s task proved even more demanding – it utilised 6,144 chips to train its 540 billion-parameter PaLM model.
The price tag for this infrastructure will shock you. A setup of just 500 Nvidia DGX A100 multi-GPU servers – the standard choice for training and running these models – costs about £79.42 million. The future looks even more expensive. Experts predict AI server infrastructure and operating costs will reach £60.36 billion by 2028.
Power consumption tells a similar story. Each Chat GPT query uses 2.9 watt-hours of electricity, which dwarfs a standard Google search at 0.3 watt-hours. Because of generative AI, data centre power demands will grow by 160 percent by 2030.
These power-hungry systems reshape data centre designs completely. Rack power densities doubled from 8kW to 17kW in just two years. Experts expect this number to hit 30kW by 2027. Chat GPT’s training pushes power consumption beyond 80kW per rack. This generates so much heat that traditional air-cooling systems fall short, and data centres must utilise liquid cooling technologies.
Data centres already use 1 to 1.5 per cent of global electricity, which will grow substantially as AI adoption accelerates throughout the decade.
Measuring Chat GPT’s Energy Consumption
Energy Consumption Factors
Several variables can affect how much energy AI chatbots use:
- Complexity of Algorithms: More complex algorithms typically require more processing power, resulting in higher energy usage.
- Volume of Interactions: The number of queries handled can significantly impact energy consumption. Higher traffic leads to more computational demand.
- Infrastructure: The efficiency of the servers and data centres hosting the chatbots plays a crucial role. Modern, energy-efficient data centres can reduce overall energy consumption.
Energy Consumption of AI Models
The energy consumption of AI models, particularly those used in chatbots, is a significant consideration. Training these models can be resource-intensive, requiring substantial computational power.
- Training: This phase involves teaching the AI model using large datasets. It is computationally expensive and can consume vast amounts of energy, sometimes equivalent to the energy usage of several households over a year.
- Inference: Once trained, the model can respond to user queries. This phase is generally less energy-intensive than training but can still contribute to overall energy consumption, especially with high volumes of user interactions.
The numbers behind Chat GPT’s energy appetite are eye-opening at every level of operation. Recent research shows that a single Chat GPT query creates between 0.3 and 4.32 grammes of CO2, although these estimates vary quite a bit across studies.
Each Chat GPT interaction uses about 2.9 watt-hours of electricity, which is ten times more than what a Google search takes. However, newer studies point to 0.3 watt-hours for typical GPT-4o queries. This amount equals running an LED bulb for just minutes.
The numbers get more significant faster with scale. Chat GPT handles about 1 billion queries daily and uses 3 million kilowatt-hours of energy daily. That’s 100,000 times more power than a typical US home needs. The yearly total hits 1,059 gigawatt-hours, matching what the entire nation of Barbados uses in a year (1,100 GWh in 2023).
This massive energy use leaves a big carbon footprint. Chat GPT creates 260,930 kg of CO2 every month. Picture 260 planes flying from New York to London—the same impact.
Scientists use two main ways to get these numbers. They look at NVIDIA server power needs since these machines run most AI systems. They also measure specific AI tasks with tools like CodeCarbon.
The way scientists measure makes a big difference in results. Model size, query length, and better hardware change the numbers. A long query (10,000 tokens) might need 2.5 watt-hours, while massive inputs (100,000 tokens) could take up to 40 watt-hours.
AI keeps growing, so these numbers will shift. The message stays clear, though – every chat with Chat GPT adds to a hefty energy bill that builds up fast.
Real-World Impact of AI Energy Usage
Chat GPT’s environmental footprint reaches way beyond simple electricity bills. AI systems continue to expand, and their combined effect on global resources becomes more apparent daily.
AI energy needs are changing power consumption patterns across the globe. Data centres use 1-2% of global power, and experts project this number to reach 3-4% by 2030. Goldman Sachs Research predicts data centre power needs will rise 160% by decade. This means an additional 200 terawatt-hours each year between 2023 and 2030.
Rising energy use leads to higher carbon emissions. Microsoft’s CO2 emissions have jumped nearly 30% since 2020. Google’s greenhouse gas output in 2023 showed an almost 50% increase compared to 2019. Data centre CO2 emissions could double between 2022 and 2030. This represents a ‘social cost’ of £99.27-140 billion.
Water usage creates another significant problem. Data centres need large amounts of water to cool their systems. Each kilowatt hour of energy requires two litres of water. A single Chat GPT conversation uses about 500 millilitres of water. Google’s data centres alone used about 5.2 billion gallons worldwide in 2022.
This growth puts unprecedented strain on regional power grids. AI could account for about 19% of data centre power needs by 2028. US data centres will use 8% of power by 2030, up from 3% in 2022. Utilities will need to invest around £39.71 billion in new generation capacity.
Power generation needs strong infrastructure support. Over the next decade, European countries with ageing power grids will need nearly €800 billion (£683.77 billion) for transmission and distribution and almost €850 billion for renewable energy investments.
Despite these challenges, AI technology offers some environmental benefits. Through climate modelling, transport efficiency improvements, and renewable energy integration, it could help alleviate 5-10% of global greenhouse gas emissions by 2030.
Number of Data Centres in the AU, EU, and UK
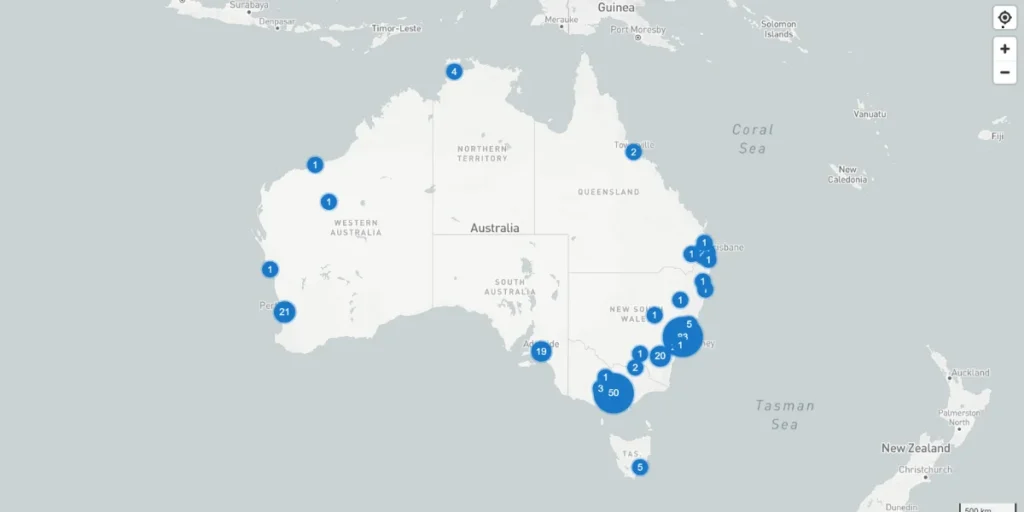
Australia: There are about 200 data centres in Australia, and sustainability and energy efficiency are becoming increasingly important. In recent developments, the use of renewable energy is becoming more prevalent.
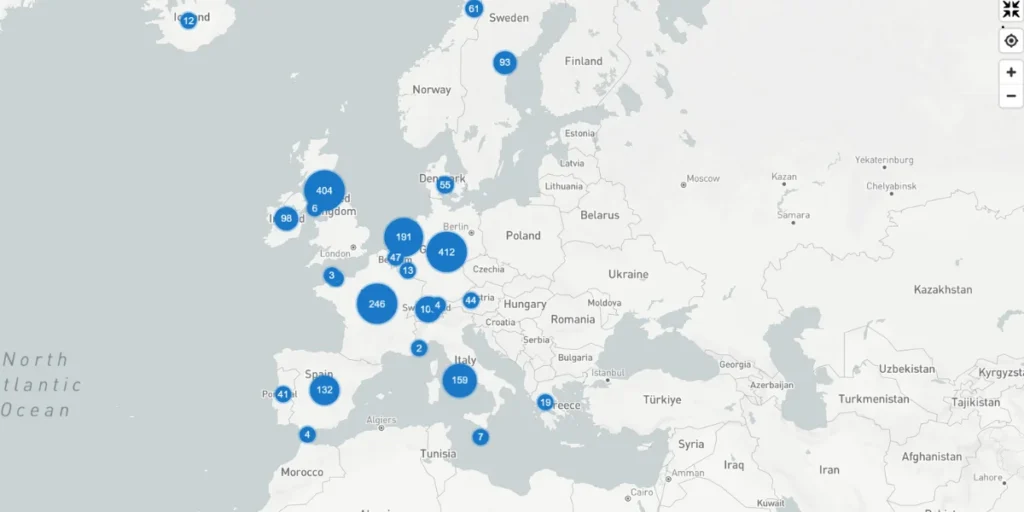
European Union: The EU has seen a surge in data centres, with over 500 facilities operating across member states. Many of these facilities use renewable energy, emphasizing sustainability and energy efficiency.
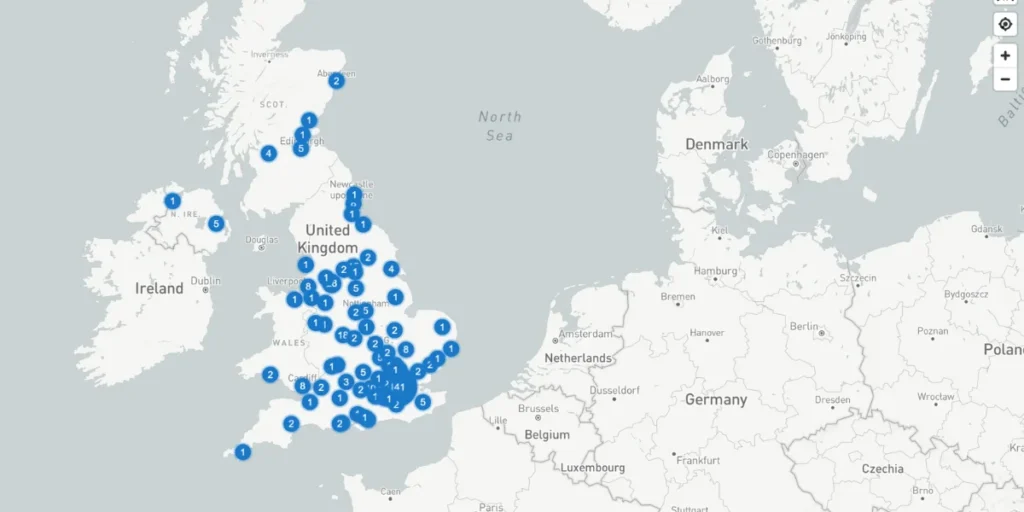
United Kingdom: The UK is home to approximately 300 data centres. The industry is rapidly evolving, strongly emphasising reducing energy consumption through innovative technologies.
Conclusion – Chat GPT Energy Consumption
Chat GPT’s widespread adoption hides serious environmental and infrastructure challenges that need immediate action. Every chat with this AI system uses 2.9 watt-hours per query, creating up to 4.32 grammes of CO2. These numbers add up quickly with billions of daily chats.
AI data centres already use 1-2% of global power. Experts predict this will jump 160% by 2030. The infrastructure needs are massive. The UK’s private sector has pledged £25 billion, while Australia plans to reach 2,500 megawatts capacity by 2033.
Environmental concerns go beyond power usage. Cooling systems need 500 millilitres of water per conversation, and data centre emissions might double between 2022 and 2030. These issues raise important questions about eco-friendly AI growth and wise resource use.
Power grids are under extreme stress and need huge upgrades in transmission infrastructure and clean energy sources. European nations must invest £683.77 billion to improve their grids and £850 billion for renewable energy projects over the next decade.
AI systems create big environmental challenges but could also help solve them. AI applications in climate modelling, transport optimisation, and renewable energy could cut global greenhouse gas emissions by 5-10% by 2030. This shows the complex relationship between environmental effects and tech progress.
How much energy does a typical ChatGPT query consume?
A typical ChatGPT query consumes approximately 0.3 watt-hours of electricity, equivalent to running an LED light bulb for a few minutes.
How does ChatGPT’s energy usage compare to other everyday activities?
ChatGPT’s energy consumption is relatively low compared to that of common household activities. For instance, 100 ChatGPT queries use the same energy as running a standard 60W light bulb for one hour.
Does using ChatGPT contribute significantly to water consumption?
Yes, ChatGPT does use water to cool its data centres. It’s estimated that each conversation uses about 500 millilitres of water, which is primarily used to cool the servers.
Are there any potential environmental benefits to using AI like ChatGPT?
While AI systems consume energy, they also have potential to contribute to environmental solutions. For example, AI could help optimise energy use, improve climate modelling, and enhance renewable energy integration.
How is the tech industry addressing the energy consumption of AI systems?
The industry is working on developing more energy-efficient hardware and algorithms. Additionally, various tech companies are investing in renewable energy sources to power their data centres, in an effort to reduce the impact AI systems have on the environment.